Data Discipline: The Foundation for AI Success
In the race to adopt artificial intelligence, many organizations overlook a critical factor: data discipline. Without it, even the most sophisticated AI tools can fall short and deliver inaccurate insights that frustrate customers and drain resources. This blog explores how data discipline underpins AI success and how you can ensure your systems are ready for the challenge.
- Why Data Discipline is the Bedrock of AI
- What is Data Discipline?
- The Risks of Ignoring Data Discipline Before Adopting AI
- What Strong Data Discipline Looks Like
- How to Assess If Your Data is Ready for AI Success
- Why Data Discipline is Non-Negotiable in the AI Era
- The First Step Toward Data-Driven AI Success
Why Data Discipline is the Bedrock of AI
AI thrives on data—but not just any data. High-quality, well-structured, and carefully governed data is essential. Think of data as fuel for AI. AI systems are only as effective as the data they process—as they say, “garbage in, garbage out.” Missteps in data management can derail your AI efforts, lead to bad decisions, alienated customers, and reputational damage.
The Risks of Ignoring Data Discipline Before Adopting AI
Skipping the groundwork of data discipline can create major roadblocks:
Inaccurate AI Predictions and Recommendations
When you feed AI systems incomplete, inconsistent, or outdated data, they produce unreliable outputs. For example, a predictive model that recommends personalized offers may suggest irrelevant products if it draws from old or incorrect customer behavior data. This can frustrate customers and lead to missed revenue opportunities.
Operational Inefficiencies and Increased Costs
Poor data management creates inefficiencies in AI deployment. Inconsistent data sources can result in duplicate efforts, misaligned processes, and higher costs due to manual intervention needed to correct errors. This makes AI adoption more expensive and time-consuming. It reduces its return on investment (ROI).
AI Bias and Unintended Consequences
Inconsistent data introduces bias into AI systems, which can lead to unfair outcomes. For example, an AI chatbot trained only on data from certain customer segments might inadvertently provide poor service to other demographics and damage your company’s reputation.
Compliance Risks and Data Security Issues
With stricter regulations like the General Data Protection Regulation (GDPR) and the California Consumer Privacy Act (CCPA), you must handle customer data carefully. Poor data discipline increases the risk of non-compliance. It leads to fines, legal challenges, and loss of customer trust. You must integrate your AI systems into a data governance framework that ensures secure data handling.
Disjointed Customer Experiences
If data isn’t well-organized across departments, different teams may act on conflicting information. This ultimately creates fragmented and inconsistent customer interactions. For instance, marketing may send a promotion to a customer flagged by the support team as dissatisfied and expose your company to a brand-damaging lack of coordination.
What Strong Data Discipline Looks Like
To fully harness AI’s potential, you’ll need to set up processes that drive data discipline and emphasize accuracy, consistency, accessibility, and governance. Here’s what a strong data discipline looks like:
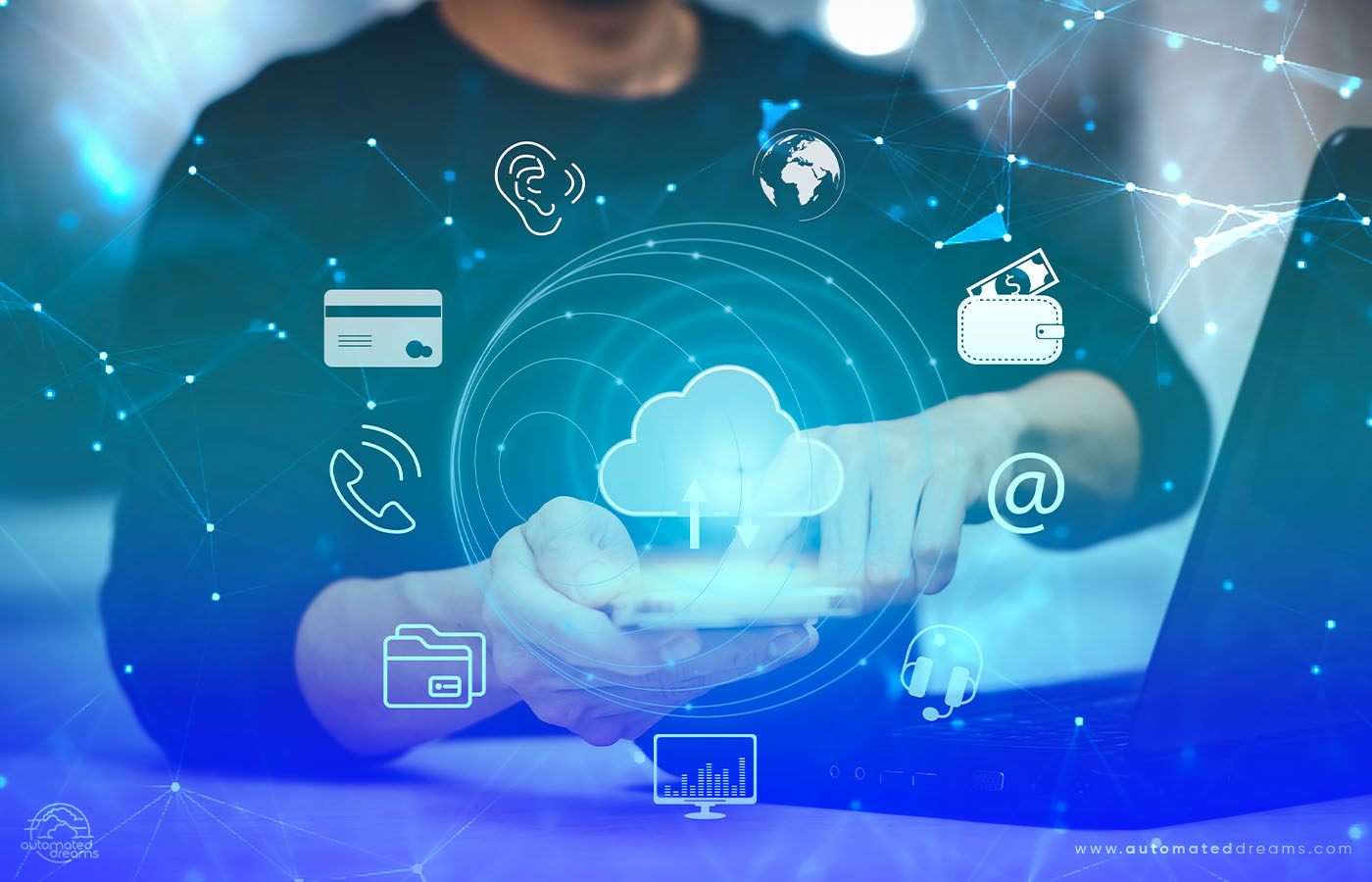
1. Centralized Data Infrastructure
Break down silos by consolidating data into platforms like Customer Data Platforms (CDPs) or data lakes. Unified systems ensure all teams have access to the same accurate and up-to-date information.
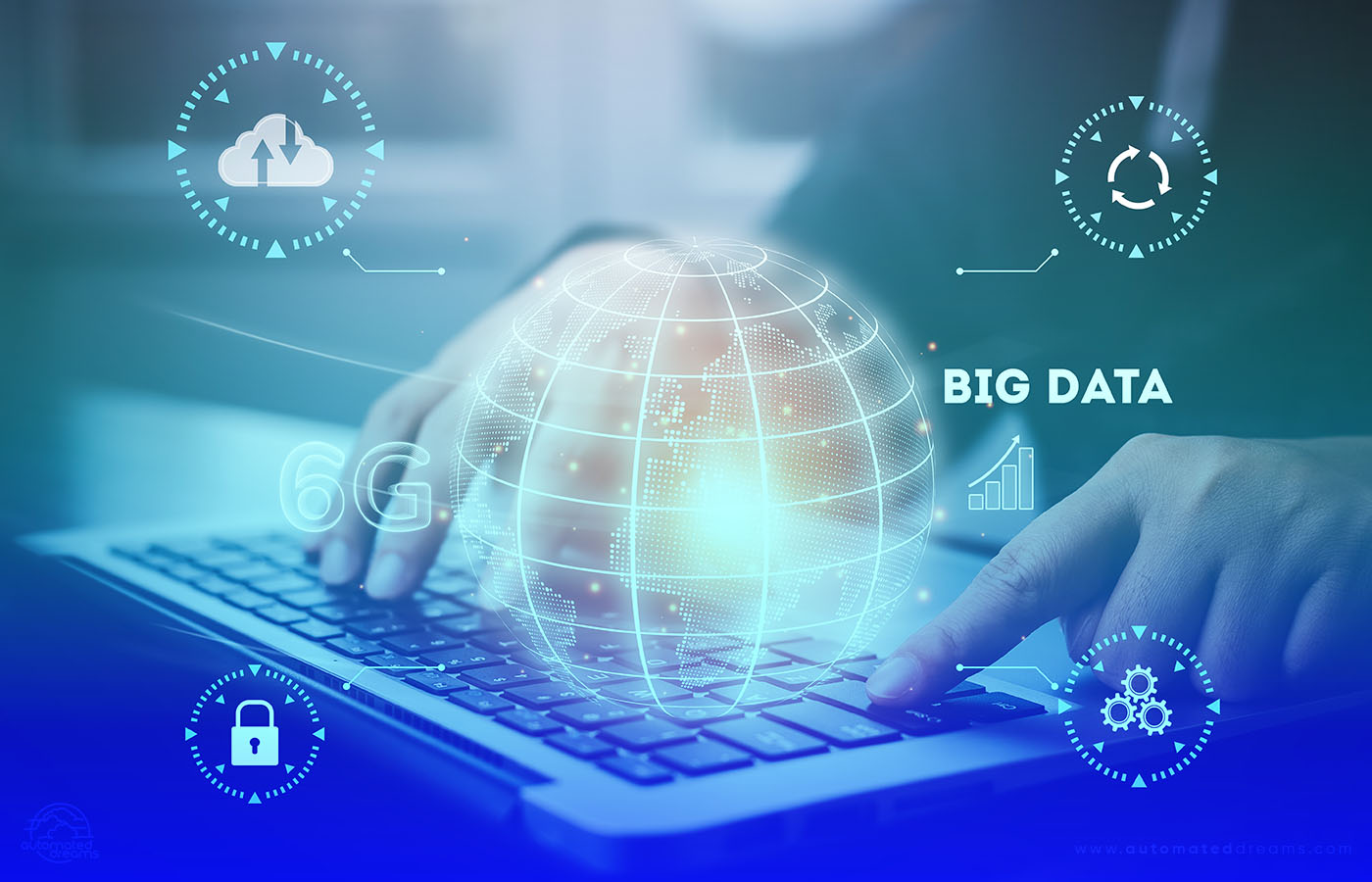
2. Rigorous Data Quality Management
Automate processes to regularly cleanse, validate, and enrich data. This includes removing duplicates, correcting errors, and ensuring consistency across all systems. Tools for automated data validation can help identify and correct inaccuracies in real time.
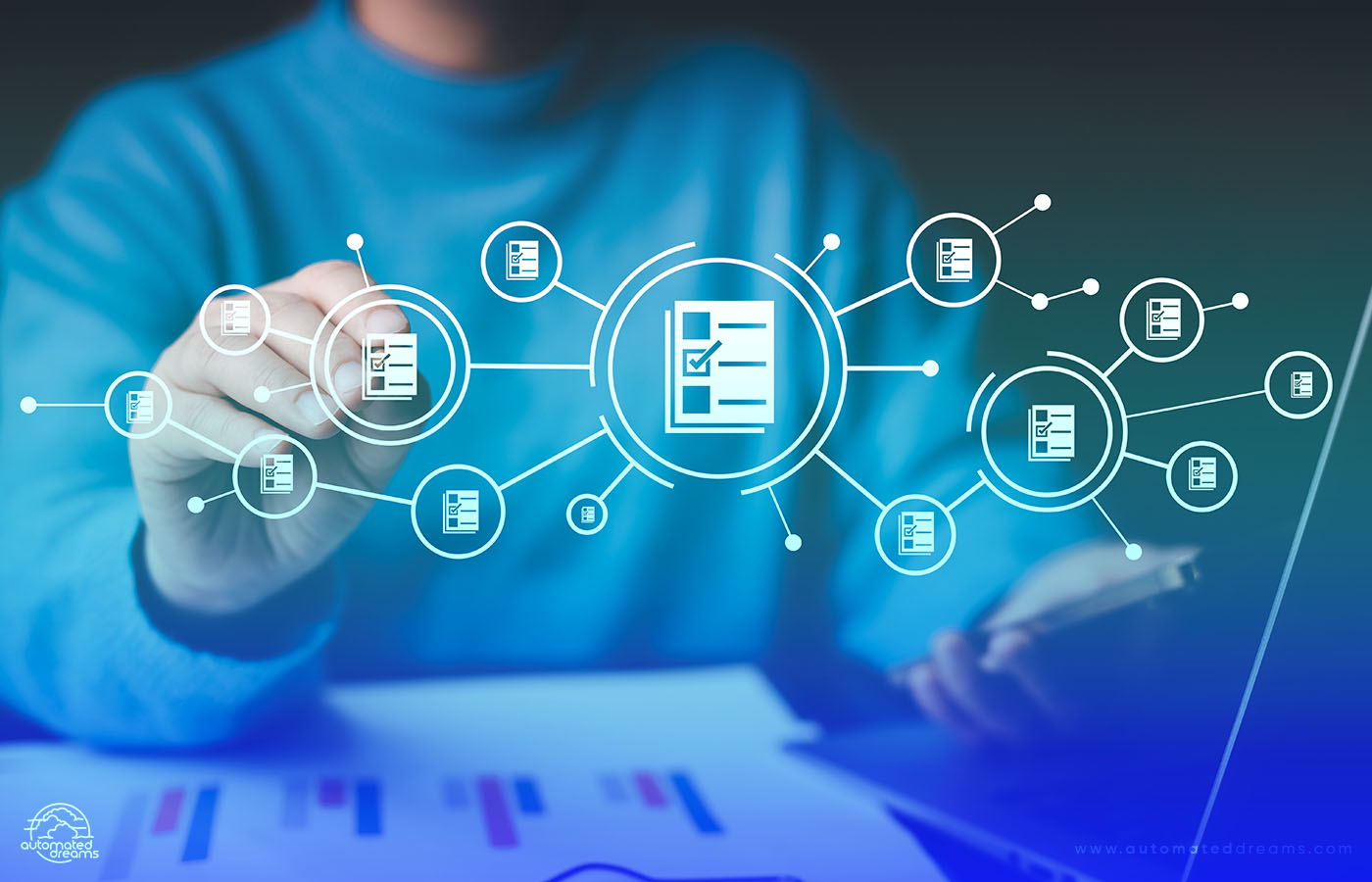
3. Clear Data Governance Framework
Create clear policies that define who owns data, how it is used, and how it flows across departments. Data governance ensures accountability and prevents misuse by setting rules for collection, storage, and usage aligned with privacy regulations.
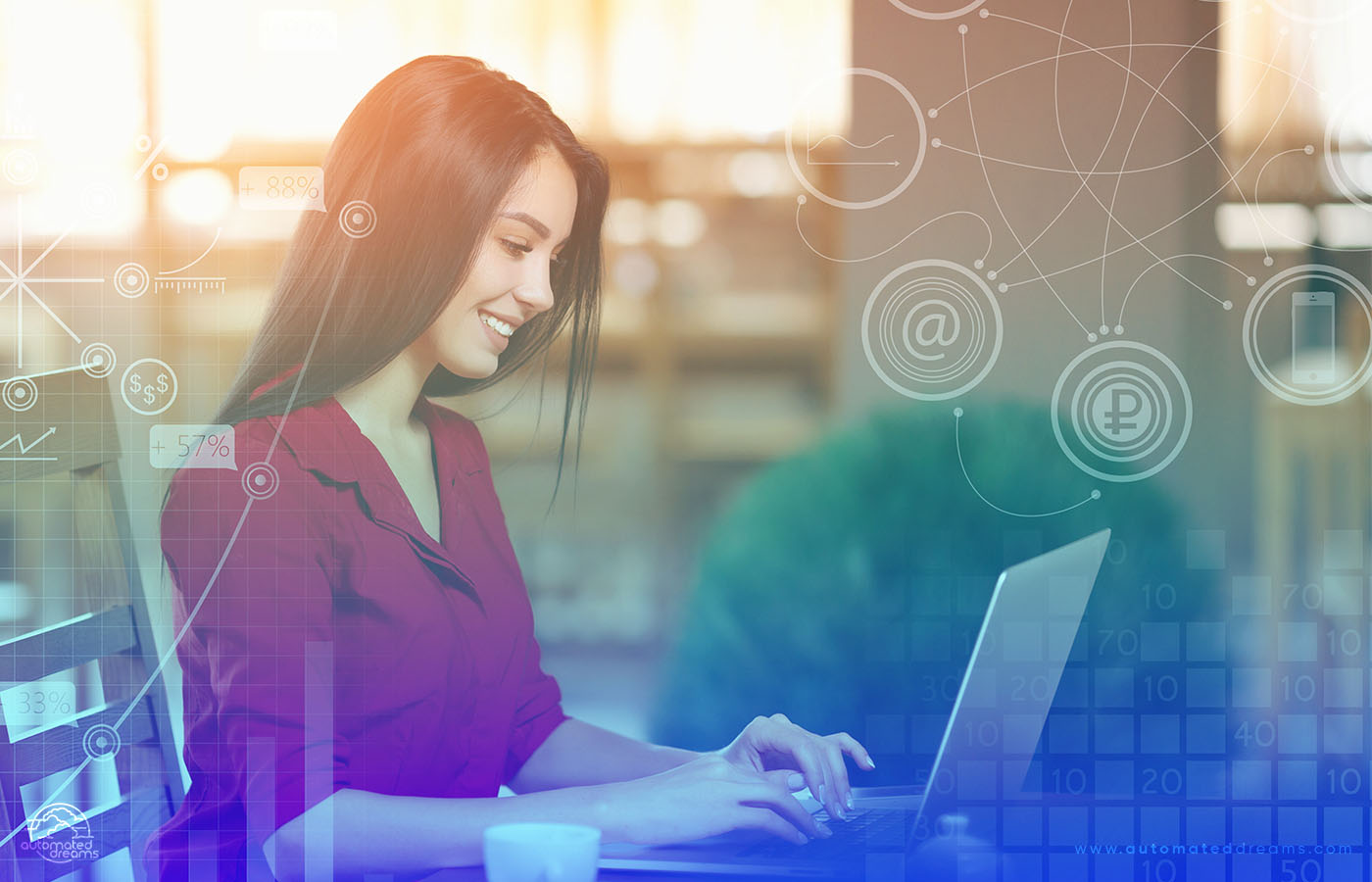
4. Accessible and Well-Structured Data
For AI to be effective, data needs to be easily accessible and structured in a way that AI models can process. Organize data into AI-friendly formats like JSON or tabular structures and tag it with relevant metadata to ensure it is easy to query and analyze.
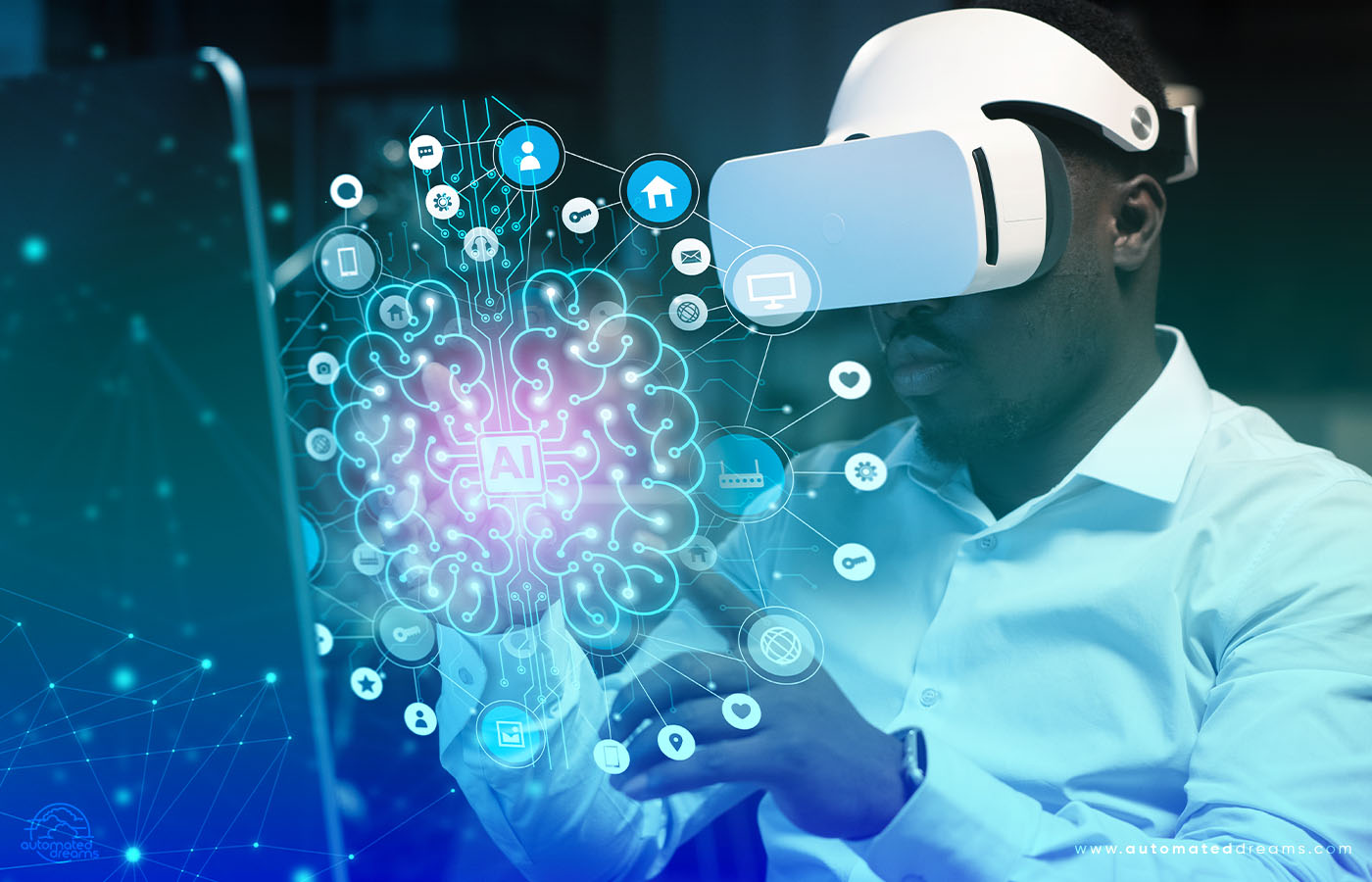
5. Real-Time Data Synchronization
AI thrives on real-time information, especially for customer-facing applications like chatbots or recommendation engines. Sync systems to avoid delays and inaccuracies across different platforms while ensuring the most recent information is available for AI-driven insights.
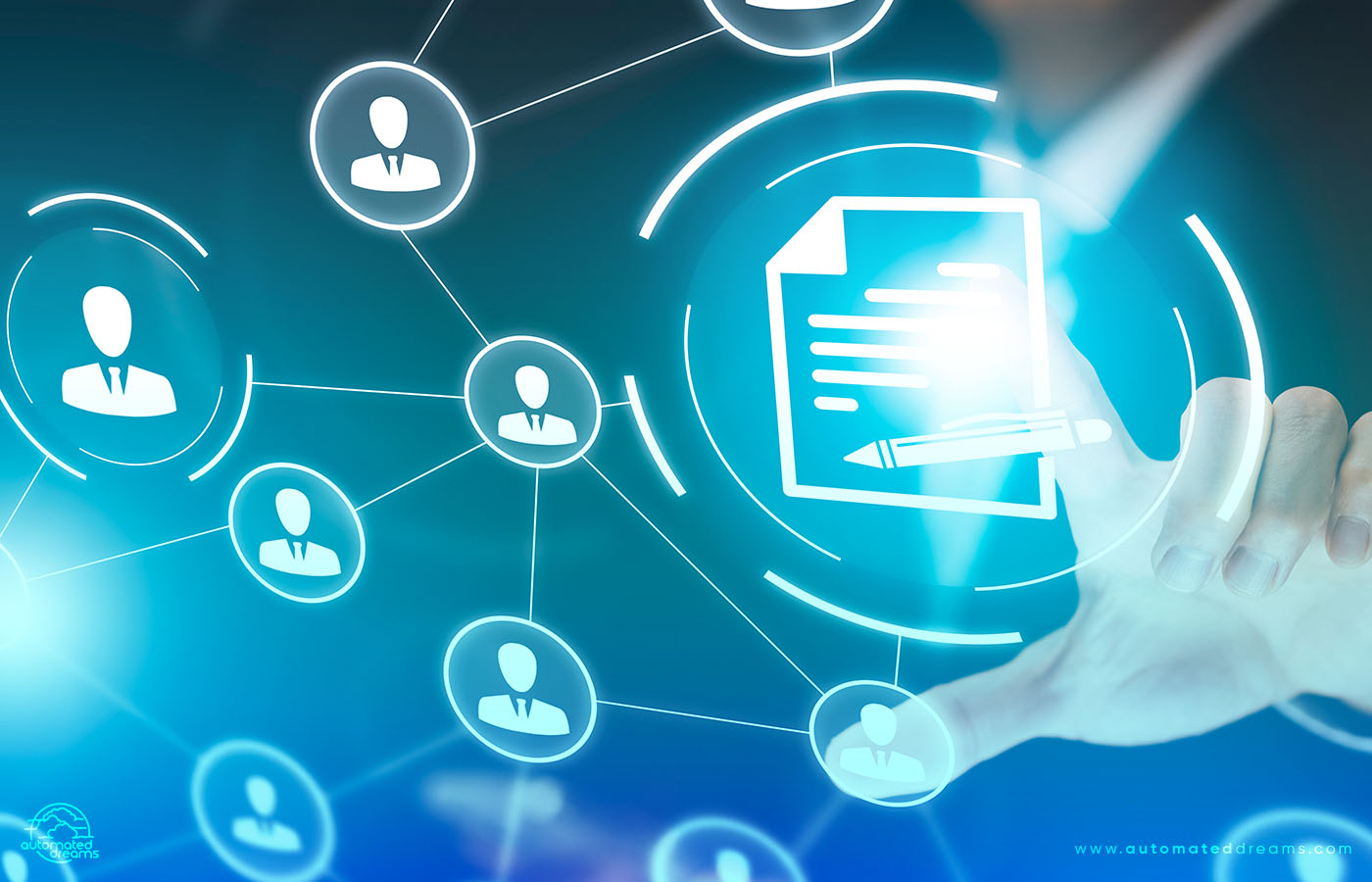
6. Employee Enablement and Training
Strong data discipline requires employees to understand the importance of data accuracy and how to manage it effectively. Invest in training your employees on data handling best practices to ensure that every team member contributes to high data quality.
How to Assess If Your Data is Ready for AI Success
Before diving into AI, you must evaluate your current data practices to determine if your data is AI-ready. Here are key questions and steps to assess data readiness:
- Do We Have Clean, Consistent Data Across Systems?
- Are there duplicate records or conflicting data points across departments?
- Has data been regularly validated and cleansed to eliminate errors?
If data is messy, AI systems will struggle to generate useful outputs.
- Is Our Data Accessible and Well-Structured?
- Can different teams access the data they need without silos or bottlenecks?
- Is data stored in structured formats that AI models can easily process (e.g., JSON, tabular data)?
If data isn’t structured or accessible, AI adoption will be slow and error-prone.
- Do We Have a Centralized View of Our Customer Data?
- Are all customer interactions and touchpoints logged in a unified platform, like a CDP or CRM?
- Can we quickly pull reports on customer behavior across channels?
AI performs best when it can access a 360-degree view of the customer.
- Are Data Governance Policies in Place?
- Do we have clear guidelines on who owns what data and how it’s managed?
- Are we compliant with data privacy regulations like GDPR or CCPA?
Governance ensures that AI-driven processes remain ethical, secure, and aligned with legal standards.
- Do We Have Real-Time Data Capabilities?
- Can our systems update data in real time?
- Are there automated processes in place for syncing data across platforms?
Real-time data is essential for AI applications that require up-to-the-moment insights.
- Are We Tracking Key Data Quality Metrics?
- Do we monitor data completeness, accuracy, timeliness, and consistency?
- Are there processes in place to quickly address data quality issues?
Tracking data quality metrics helps to identify and solve issues before they impact AI performance.
Why Data Discipline is Non-Negotiable in the AI Era
Building strong data discipline is an ongoing process, not a one-time effort. It requires collaboration across departments, continuous monitoring, and a culture that values data quality. Companies that take the time to evaluate and improve their data practices will find themselves better positioned to implement AI successfully, drive meaningful improvements in customer experience, and maintain a competitive edge in their industry.
Businesses that skip this step risk wasting time and resources on AI projects that fail to deliver value.
The First Step Toward Data-Driven AI Success
Ready to take your data practices to the next level? Begin by auditing your current data quality and governance frameworks. Implement tools that centralize, clean, and validate your data. Most importantly, build a culture of accountability—because data discipline is everyone’s responsibility.
Prioritize data discipline in AI, and watch your systems transform into engines of growth, precision, and innovation. Your journey to AI excellence starts here.